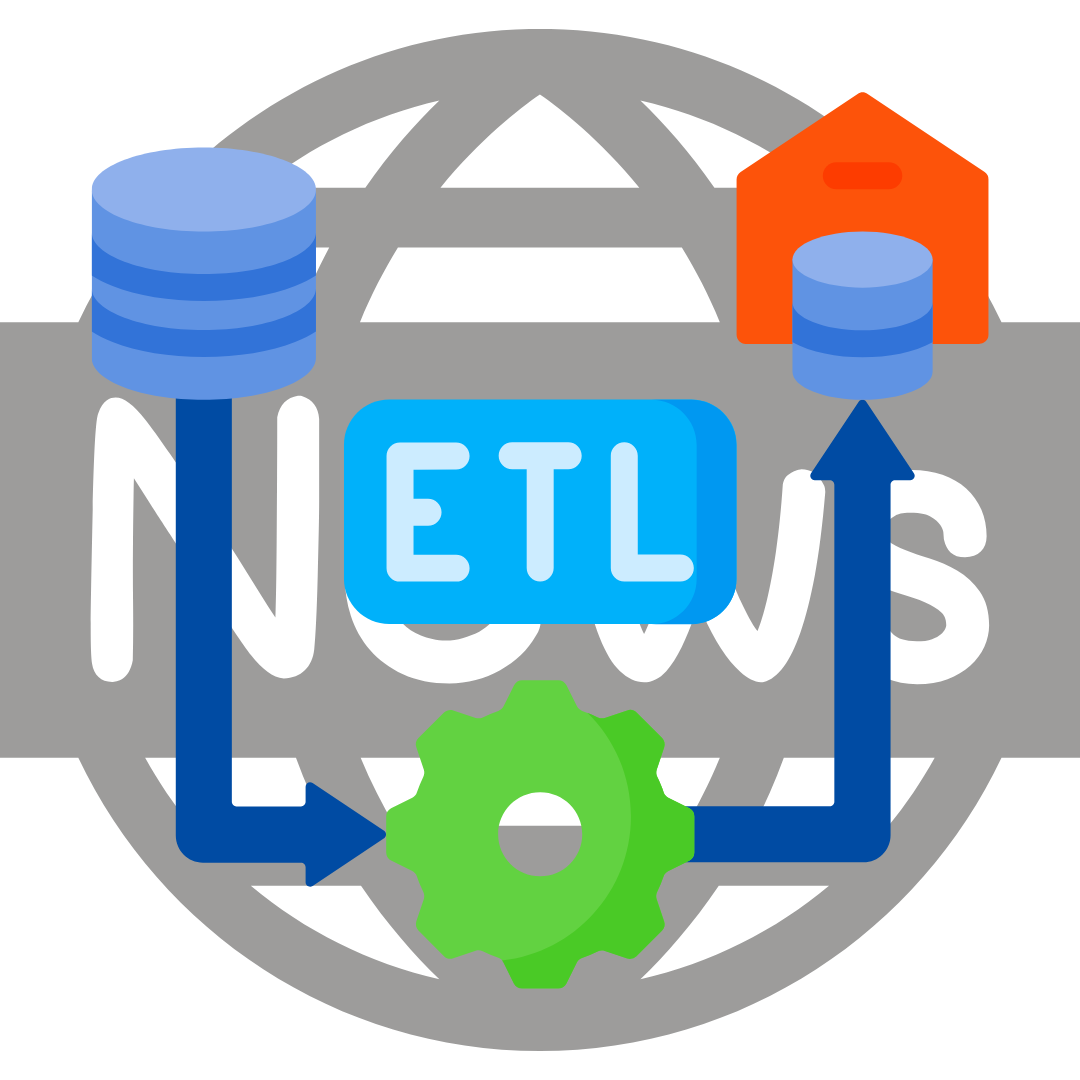
Landslide Segmentation Using U-Net and Satellite Imagery
Landslide Segmentation Using U-Net and Satellite Imagery
This project implements a landslide segmentation model using a U-Net architecture. The goal is to predict segmentation masks for landslide-prone areas based on satellite imagery. The project includes preprocessing of .h5
files containing the images, training a U-Net model using PyTorch, evaluating the model, and finally deploying the model using a Streamlit app for inference on user-uploaded images.
Steps Performed
Dataset Loading & Visualization:
- Satellite images and their corresponding masks are stored in .h5 format.
- h5py is used to read and visualize the dataset. The images consist of multiple channels including RGB, NIR (near-infrared), NDVI (Normalized Difference Vegetation Index), slope, and elevation.
- Sample images and masks are displayed using matplotlib
- NDVI Calculation
- NDVI is computed from the NIR and Red bands of the satellite images
Data Preprocessing:
- The dataset is preprocessed by normalizing the RGB, slope, and elevation channels.
- Any NaN values in the dataset are replaced with small values (e.g., 0.000001).
Dataset Splitting:
- The dataset is split into training and validation sets using
train_test_split
fromsklearn
. - Data is then structured into PyTorch
Dataset
andDataLoader
objects to facilitate batching during training.
- The dataset is split into training and validation sets using
Model Architecture (U-Net):
- A U-Net model is defined with multiple convolutional and downscaling blocks (
DoubleConv
,Down
,Up
). - The model is designed to take 6-channel inputs and output a binary segmentation mask.
- The U-Net architecture is implemented using PyTorch and includes dropout regularization to prevent overfitting.
- A U-Net model is defined with multiple convolutional and downscaling blocks (
Loss Function:
- A combination of binary cross-entropy loss and Dice loss is used for training.
- Dice loss helps improve performance on imbalanced datasets by focusing on overlapping areas between predicted and true masks.
Training Loop:
- The model is trained for 30 epochs using the Adam optimizer and the combined loss function.
- Training and validation losses are recorded, and the model with the lowest validation loss is saved as
best_model.pth
.
Model Evaluation:
- After training, the model is evaluated using accuracy, precision, recall, and F1 score metrics.
- Predictions are generated on the validation set, and these metrics are computed by comparing predicted masks with ground truth masks.
Visualization:
- A random sample from the validation set is selected, and its input image, true mask, and predicted mask are visualized side by side using
matplotlib
.
- A random sample from the validation set is selected, and its input image, true mask, and predicted mask are visualized side by side using
Our Latest Projects
Far far away, behind the word mountains, far from the countries Vokalia and Consonantia
About
An AI Geek and a lifelong learner, who thrives in coding and problem-solving through ML, DL, and LLMs.
Copyright ©2024 All rights reserved.